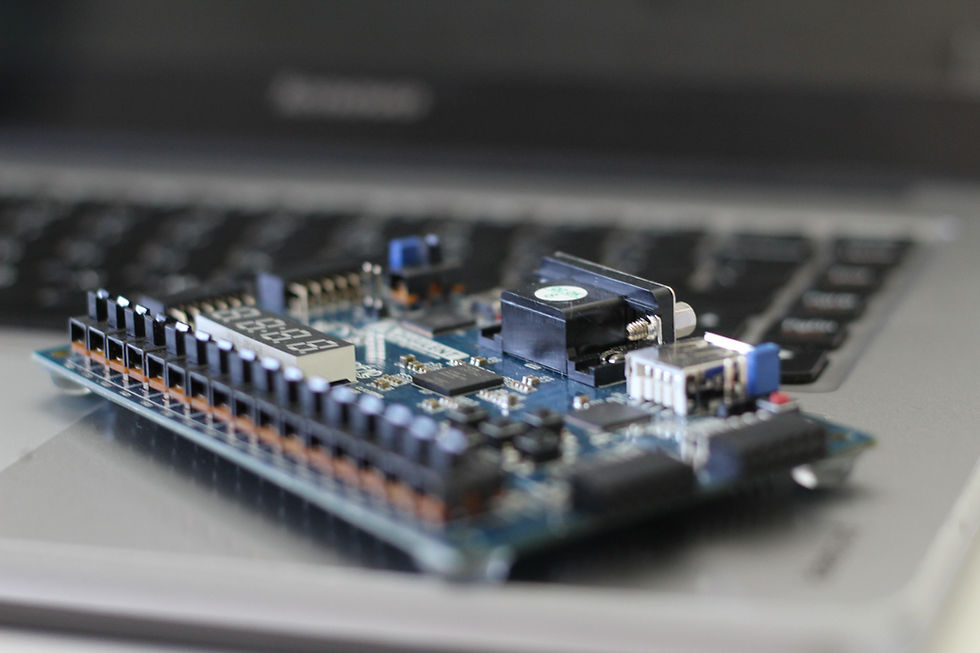
There could be a new era of co-design dawning for machine learning, one that moves away from the training and inference separations and toward far less dense networks with highly sparse weights and activations. While 2020 was peak custom AI chip, it could be that the years ahead feature devices we already know but with re-imagined circuits that get us closer to that brain-like efficiency, performance, and continuous learning that has been the golden grail of AI since the beginning.
There could be nothing further from human brain function in learning than what we have now: dense networks, unpacked in batches on massive matrix multiplication units that are then fed into a separate, albeit lower-power capable inference phase. While neuroscience is often the touted inspiration for almost all we’ve heard from the AI chip startups and established accelerator vendors over the years, the hardware doesn’t meet the theory in the middle.
Emphasizing sparsity, both in terms of devices and frameworks, has been another area where there has been plenty of lip service but ultimately, especially for accelerators that want to do both training, inference, and general compute workloads cannot be fit into the sparsity mold—for good reasons. But if the training and inference steps are no longer separate, if learning is continuous and doesn’t need to conform to the batch-driven notion of “forgetting” what it’s learned to move on to something new, a truly new paradigm opens for machine learning.
Read more at:
/Service Ventures Team
Comments