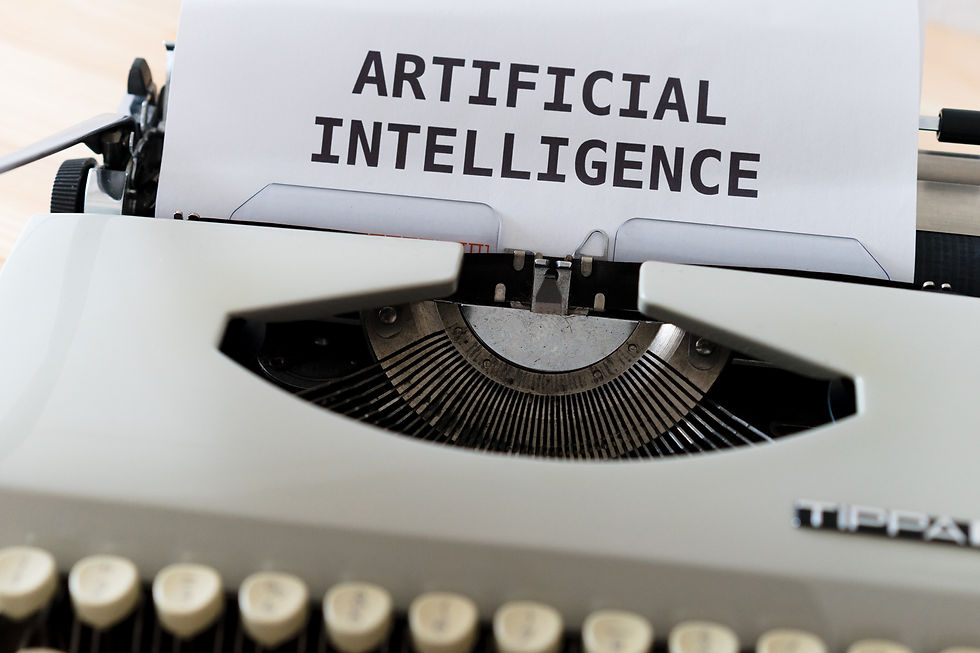
Defining Artificial Intelligence (AI)
Artificial Intelligence (AI) is a general computer science term that refers to Hardware or Software that exhibit human like intelligent behavior. Basic AI concepts have existed since the 1950s, via rules-based programs that display rudimentary intelligence in limited contexts. Early forms of AI included ‘expert systems’ designed to mimic human specialists. But rules-based systems are limited. Many real-world challenges, from making medical diagnoses to recognizing objects in images, are too complex or subtle to be solved by programs that follow sets of rules written by people.
However, recent excitements regarding modern AI relates to a set of new techniques called Machine Learning (ML) that is seeing very rapid, significant technical advances and creating the hope that there could be the possibility to create human like smart systems that could compliment human beings in carrying out more complex analysis of the real world problems that are not possible with the current computer systems/solutions. Machine Learning (ML) is a sub-set of AI i.e. all Machine Learning is AI, but not all AI is Machine Learning. Machine learning enables programs to learn through training, instead of being programmed with rules. This is achieved by first training a SW program through the processing of a large set of pre-defined training (Training) data in a controlled manner and allowing the SW to create partial ‘learnings’ about a challenge. Then the SW is presented with unknown real data that needs to be analyzed and the SW infers (Inference) and provides insights to humans based on earlier ‘learnings’ achieved by the SW during the training process.
Machine Learning (ML) can be applied to a wide variety of prediction and optimization challenges, from determining the probability of a credit card transaction being fraudulent to predicting when an industrial asset is likely to fail. There are several approaches to machine learning. Popular methodologies include random forests, Bayesian networks and support vector machines. Deep Learning (DL) is a subset of machine learning that is of high interest and is delivering breakthrough results in fields including computer vision, natural language processing and many other uses. All Deep Learning (DL) is Machine Learning (ML), but not all Machine Learning is Deep Learning. Deep learning emulates the way human brains learn subtle tasks – it models the brain, not the world. Networks of artificial neurons process input data to extract features and optimize variables relevant to a problem, with results improving through the training process.
Why We Think AI is Important
AI technology is important because it enables human capabilities – understanding, reasoning, planning, communication, and perception – to be undertaken by software increasingly effectively, efficiently and at low cost. General analytical tasks, including finding patterns in data, that have been performed by software for many years can also be performed more effectively using AI. The automation of these abilities creates new opportunities in most business sectors and consumer applications. Significant new products, services and capabilities enabled by AI include autonomous vehicles, automated medical diagnosis, voice input for human-computer interaction, intelligent agents, automated data synthesis and enhanced decision-making.
AI has numerous, tangible use cases today that are enabling corporate revenue growth and cost savings in existing sectors. Applications will be most numerous in sectors in which a large proportion of time is spent collecting and synthesizing data: financial services, retail and trade, professional services, manufacturing, and healthcare. Applications of AI-powered computer vision will be particularly significant in the transport sector. Use cases are proliferating as AI’s potential is understood. There are many use cases across various sectors such as asset management, healthcare, insurance, law & compliance, manufacturing, retail, transportation, and utilities.
Why We Think AI can Succeed Now
After several false dawns since its inception in 1956, AI technology has come of age. The capabilities of AI systems have reached a tipping point due to the confluence of seven factors: new algorithms, the availability of training data, specialized hardware, cloud based elastic AI services, open-source software resources, greater capital investment, and increased interest from end users. Together, these developments have transformed results while slashing the difficulty, time, and cost of developing and deploying AI. A virtuous cycle has developed - progress in AI is attracting investment, entrepreneurship, and interest. These, in turn, are accelerating further progress in the field.
Current State of AI Adoption
In 2019, AI ‘crosses the chasm’ from early adopters to the early majority. We believe AI is the fastest paradigm shift in technology history. AI adoption has tripled in last 12-18 months. Reports show that one in seven large companies has adopted AI; in next 24 months, two thirds of large companies will have live AI initiatives, in next three years, the proportion of enterprises with AI initiatives will have grown from one in 25 to one in three. Adoption has been enabled by the prior paradigm shift to cloud computing, data analytics, the availability of plug-and-play AI services from global technology vendors and a thriving ecosystem of AI-led software suppliers. Great expectations are fueling adoption. Executives expect AI to have a greater impact than any other emerging technology, including Blockchain and Quantum. Increasing adoption is also masking a growing divergence between leaders and laggards - Leaders are extending their advantage by learning faster and increasing investment in AI at a greater pace than laggards.
Twice as many enterprises in Asia have adopted AI, compared with companies in North America, due to government initiatives and engagements, a data advantage and fewer legacy assets. But we also see sector adoption uneven and in a state of flux. ‘Early adopters’ (Financial. High-Tech) maintain a lead while ‘movers’ (Retail, Healthcare, Media) are rapidly catching up. Manufacturing, Industrial, and Energy are laggards in AI adoption. AI is advancing across a broad front. Enterprises are currently using multiple types of AI application, with one in ten enterprises using ten or more. The most popular use cases are chatbots, process automation solutions and fraud analytics. Natural language processing and computer vision AI underpin many prevalent applications as companies embrace the ability to replicate traditionally human activities in software for the first time.
Leaders and laggards face different adoption challenges. Laggards are struggling to gain leadership support for AI and to define use cases. Leaders’ difficulties, in contrast, have shifted from ‘if’ to ‘how’. Leaders are seeking to overcome the difficulty of hiring talent and address cultural resistance to AI. AI initiation has shifted from the C-suite to Lines of Business (LoBs) and IT department. Two years ago, CXOs initiated two thirds of AI initiatives. In 2019, as corporate engagement with AI shifts, the LoBs and IT department the primary driver of projects. Companies prefer to buy external AI solutions. Most companies will favor buying AI solutions from third parties, while a few cutting-edge enterprises may intend to build custom solutions. Workers expect AI to increase the safety, quality, and speed of their work. We notice that as companies’ AI agendas shift from revenue growth to cost reduction initiatives, workers are concerned about job security
The Multitude Advancements in AI
While graphical processing units (GPUs) catalyzed AI development in the past, and will continue to evolve, hardware innovations are expanding AI’s potential. Hardware is being optimized, customized, or re-architected to deliver a new generation of AI compute accelerators. AI involves a heavy dose of matrix multiplication called tensor processing. Hardware with ‘tensor architectures’ is accelerating deep learning AI. Vendors, including NVIDIA and Google are optimizing or customizing hardware to support the use of popular deep learning frameworks. Silicon industry has entered the post-GPU era. Leading hardware manufacturers are creating new classes of computer processor designed, from inception, for AI. Custom silicon offers transformational performance and greater versatility due to their AI specific capabilities. Power efficient custom silicon is also taking AI to the ‘edge’ of the internet – to IoT devices, sensors, and vehicles. New processors engineered for edge computing combine high performance with low power consumption and small size. Processing data at the edge for AI has many advantages such as low latency, low BW cost, faster decisions, data sovereignty.
As quantum computing matures, it will create profound opportunities for progress in AI and enable humanity to address previously intractable problems, from personalized medicine to climate change. While nascent, quantum computing is advancing rapidly. Researchers have developed functioning neural networks on quantum computers.
Reinforcement learning (RL) is an alternative approach to developing AI that enables a problem to be solved without knowledge of the domain. Instead of learning from training data, RL systems reward and reinforce progress towards a specified goal. AlphaGo Zero, an RL system developed by DeepMind to play the board game Go, developed unrivalled ability after just 40 days of operation. Progress in RL is significant because it decouples system improvement from the constraints of human knowledge. RL is well suited to creating agents that perform autonomously in environments for which we lack training data.
The new research area in AI is Transfer learning (TL). TL enables programmers to apply elements learned from previous challenges to related problems. TL can deliver stronger initial performance, more rapid improvement, and better long-term results. Interest in TL has grown seven-fold in 24 months and is enabling a new generation of systems with greater adaptability. By learning fundamental properties of language, TL powered models are improving the state of the art in language processing – in areas of universal utility. 2018 was a breakthrough year for the application of TL to language processing. TL is also enabling the development of complex systems that can interact with the real world; delivering systems with greater adaptability; and supporting progress towards artificial general intelligence, which remains far from possible with current AI technology.
Advancements in another AI area called Generative Adversarial Networks (GANs) will reshape content creation, media, and society. An emerging AI software technique, GANs enable the creation of artificial media, including pictures and video, with exceptional fidelity. GANs will deliver transformational benefits in sectors including media and entertainment, while presenting profound challenges to societies from ‘deep fakes’.
The Big Talent Crunch
Demand for AI talent has been high. There is a gulf between demand and supply, with two roles available for every AI professional. The pool of AI talent remains small. AI demands advanced competencies in mathematics, statistics, and programming; AI developers are seven times more likely to have a Doctoral degree than other developers. Supply is increasing – machine learning has become the top emerging field of employment in the United States. Greater supply is being driven by high pay, the inclusion of AI modules in university computer science courses, companies’ investment in staff training, and AI technology companies ‘pump priming’ the market with free educational resources. Over time, AI tools offering greater abstraction will make AI accessible to less specialized developers.
Talent shortages are driving the high salaries. AI professionals are among the best paid developers and their salaries continue to increase; half enjoyed salary growth of 20% or more in the last three years as winners and losers are emerging in the war for talent. The technology and financial services sectors are absorbing 60% of AI talent because these verticals are early adopters of AI technology. The current ‘brain drain’ from academia to industry is real and will have mixed implications, catalyzing AI’s immediate impact while inhibiting teaching and moving value from the public domain to private companies. High job satisfaction is intensifying the war for talent. Three quarters of AI professionals are satisfied in their current role. To optimize hiring and retention, companies must align roles to AI professionals’ primary motivators – learning opportunities, office environment and access to preferred technologies.
The Macro Implications of AI
AI will provide profound benefits to societies, including improved health, greater manufacturing, and agricultural capability; broader access to professional services; more satisfying retail experiences; and greater convenience. AI’s has many benefits such as: Innovation (new products and services); Efficacy (perform tasks more effectively); Agility/Speed (complete tasks more quickly); and Scalability (free activity from the constraints of human capacity). These benefits will have profound implications for consumers, companies, industries, and societies. By automating capabilities previously delivered by human professionals, AI will reduce the cost and increase the scalability of services, broadening global participation in markets including healthcare and transport. In multiple sectors including insurance, legal services and transport, AI will change where, and the extent to which, profits are available within a value chain. New commercial success factors – including ownership of large, private datasets and the ability to attract data scientists – will determine a company’s success in the age of AI.
New platforms, leaders, laggards, and disruptors will emerge as the paradigm shift to AI causes shifts in companies’ competitive positioning. AI-as-a-Service consumption, and subscription payment models will obviate select business models and offer new possibilities in sectors including transport and insurance. As AI gains adoption, the skills that companies seek, and companies’ organizational structures, will change. By reducing the time required for process-driven work, AI will accelerate innovation.
AI also presents significant challenges and risks. AI-powered automation may displace jobs. AI will enable the automation of certain occupations that involve routine. In other occupations, AI will augment workers’ activities. Social dislocation, with political consequences, may result. Biased systems could increase inequality. Data used to train AI systems reflects historic biases, including those of gender and race. Artificial media may undermine trust. New AI techniques enable the creation of lifelike artificial media. While offering benefits, they enable convincing counterfeit videos. Artificial media will make it easy to harass and mislead individuals and weaken societies by undermining trust. AI offers trade-offs between privacy and security. AI enables the high-tech surveillance state, with greater powers for control. China is combining real-time recognition with social scoring to disincentivize undesirable activity. Autonomous weapons may increase conflict.
Our Recommended Playbook for AI Startups
Time has come to make good on the original promise and prove that AI startups can become formidable companies, with long term differentiation and defensibility. We think that AI entrepreneurs’ key challenges will be the availability of talent, access to training data and the difficulty of creating production-ready technology that gives business RoI. AI startups should address a business function or sector (‘vertical’) and stay away from providing a ‘horizontal’ AI platform/technology. In enterprise use cases, Healthcare, Financial services, Retail and Media & Entertainment will be well served by AI startups immediately. In sectors such as Manufacturing and Agriculture, current entrepreneurial activity is modest although we think they offer larger market opportunities. Health & wellbeing is a focal point for AI entrepreneurship; more startups focus on the sector than any other. Currently a quarter of new AI startups are consumer companies, as entrepreneurs address or circumvent the ‘cold start’ data challenge. Many of these startups focus on finance or health & wellbeing. In the coming decade, developers will have a greater impact on the future of healthcare than doctors.
The big problem everyone was afraid about a few years ago — acquiring all the data necessary to train data-hungry AI algorithms has turned out to be more tractable than anticipated. Although Google and Facebook have a formidable training data advantage, a startup does not need all the data in the world, just the data necessary to solve the specific problem it is going after (if it is defined narrowly and/or vertically enough). AI startups will need to hustle and figure out data acquisition with different strategies, from partnering with data-rich institutions (e.g., hospitals for radiology images) to creating their own datasets (either synthetically or manually by spinning up and down entire teams, often overseas). AI’s root is in academics and commercializing AI solutions for industry use cases will take slightly different evolutionary path. The reason for the slow scale is AI is a deep tech and building the business will be capital heavy compared to other SW startups. There will be no lean AI startup as iterations are not speedy. We envision three types of Enterprise AI startups emerging on the horizon:
AI Infrastructure Startups: Startups providing AI tools, infrastructure (Software and Hardware) and platforms, both to other startups and established enterprises. This is a crucial layer and is located below in the AI stack w.r.t. end user.
AI-First Startups: Startups whose product simply could not function without AI at its core, whether they serve consumers or enterprises. AI is the core value proposition and is in front, left and center for their differentiated offerings. These startups will take a vertical specific lens and provide an end-to-end solution focused on a particular use case.
AI-Enabled Startups: Startups that use AI as part of a broader product or technology stack. These startups will play in a complementary way to an existing solution and will try to add value by brining in AI capabilities on top of existing solutions in the current ecosystem.
Growing the businesses of AI startups will not be easy as is evident by conditions of some of the startups that started 3-4 years ago. Certainly, some of those startups have been phenomenally successful raising large amounts of money, and consequently hired a lot of people, which is certainly one measure of scaling. Good ML engineers continue to be in short supply. Data acquisition, although tractable, still takes a lot of time and effort. Training models to an acceptable level of performance also takes time. TensorFlow may be open sourced but deploying it as scale still requires rare expertise. From a fundraising perspective, AI startups will often have an investment profile that is closer to their deep tech cousins in Semiconductor, Space Tech and Biotechnology. AI startups will have a lot of R&D to figure out before they are even able to ship a real AI product, so they are going to be behind their nimbler peers at each round, in terms of business metrics. They will show up at the Series A with a couple of pilots. At the Series B, they might have product market fit, but an immature GTM machine. Services will be an integral part of their offering as their enterprise customers are still figuring out how to use the AI based solution. Early-stage Investors will have some time to adjust to this reality and will evolve their investment criteria. Later stage investors, who are typically more metrics oriented will slowly get the new paradigm and try to figure out what to make of them. A lot will be decided by marketing noise around the deep tech as the deep tech narrative may carry some startups further. For some AI startups, it will be much harder to avoid comparisons with regular Enterprise SW/SaaS companies.
Just like any deep tech companies, AI startups are exposed to the “science project” risk and it is important to not get stuck in R&D mode for too long. Part of it will come with the DNA of the founding teams. To build an interesting AI startup, you need a lot of smart engineers who like to conclusively solve hard technical problems. Progress in AI is often capricious and resists clear timelines. We often hear people talk about how they got most of the way (say 80% accuracy) in a matter of weeks or months, but then got stuck, sometimes for years, trying to get to 90% or 95%. Sometimes startups, frustrated by the extended plateau, will spend months of efforts on a completely different approach, only to end up with a tiny improvement. Or even worse, sometimes they see the performance of the AI degrade, for example as a result of adding new datasets. Bottom line, founders often have no real idea when the next breakthrough in their AI product will happen (which is why no one has a clue when Level 5 autonomous driving will happen). So, it is often easy, even for commercially minded teams, to slip into extended R&D loops. To avoid R&D loop, startups need to build V1 of the product that has most of the intended functions and have some level of core AI. It is great in terms of getting real and early feedback from customers. Gradually the startup can insert a better version of the AI core with more refinements. Avoiding this extended loop is not easy, but ultimately, it is a question of leadership to manage shipping timelines and the cash in the bank.
For all the amazing progress of the last few years, AI is still a very imperfect technology that fails very often. This is particularly obvious (and problematic) in cases where it needs to perform at 100% accuracy (self-driving cars, medical diagnosis). Unfortunately, due to the hype, customers expect the AI to be superhuman and have essentially no tolerance for error once a machine is involved. To make matters worse, humans involved in the process often give the AI wrong instruction, causing it to fail, but customers still blame the machine. Humans in the loop do not scale in startup world but humans are often going to be in the mix to make AI work in the real world in the early phases. Startups, instead of building their own little armies of people behind the scenes to work alongside the AI, should use their customers (not own employees) to be the “humans in the loop”. Google has been doing this for decades now for their AI products. We the users of Google all spend time telling Google Photos that yes, this is the same kid in those two pictures. Google’s computer vision system could not figure it out, but we the end users are happy to tell Google and do our bit make Google algorithms better. A big part of this machine-human loop is for the AI to communicate that it has failed clearly and early, before users get frustrated or, worse, before real damage is done. There needs to be a clear workflow handover for humans to take over. Simple GUIs, like buttons offering the human multiple choices, have proven surprisingly efficient from that perspective.
AI startups should master the social dynamics of AI to ensuring wide adoption of AI, especially in the enterprise. Humans will view AI with some level of suspicion, and the trick is AI startups must empower, rather than threaten, employees. Putting AI and humans in competing mode during early adoption will not work. But putting humans in a position where they can correct and improve the AI works much better, from a social adoption perspective. It will work well when the AI solution has been built with transparency and AI-Explainability in mind.
We also believe that AI itself should not be the sole driver of value for the product. There are often several low hanging fruits that are much easier to build than the AI itself. Those can include anything from simple features, connectors to other systems, integration with payment systems or “RPA” style automation for repetitive tasks. Those integrations can matter just as much to the end user than all the AI powered part. As we mentioned earlier, the risk for AI startups is to get stuck for too long in “deep tech” mode, with an engineering-heavy team and miss all the milestones that will make the company appealing to VCs. There is typically an opportunity to build “good enough” AI that can be supplemented and enhanced by simpler SW and RPA type features and distributed efficiently through tried-and-tested marketing and sales methods, if the relevant teams are onboarded early enough. If startups can do the above, we believe that AI startups will truly scale and emerge as category defining companies. We are still early in this wave of creation of AI startups with many more use case opportunities, and we are excited to see how it all plays out.
/Service Ventures Team
Comments