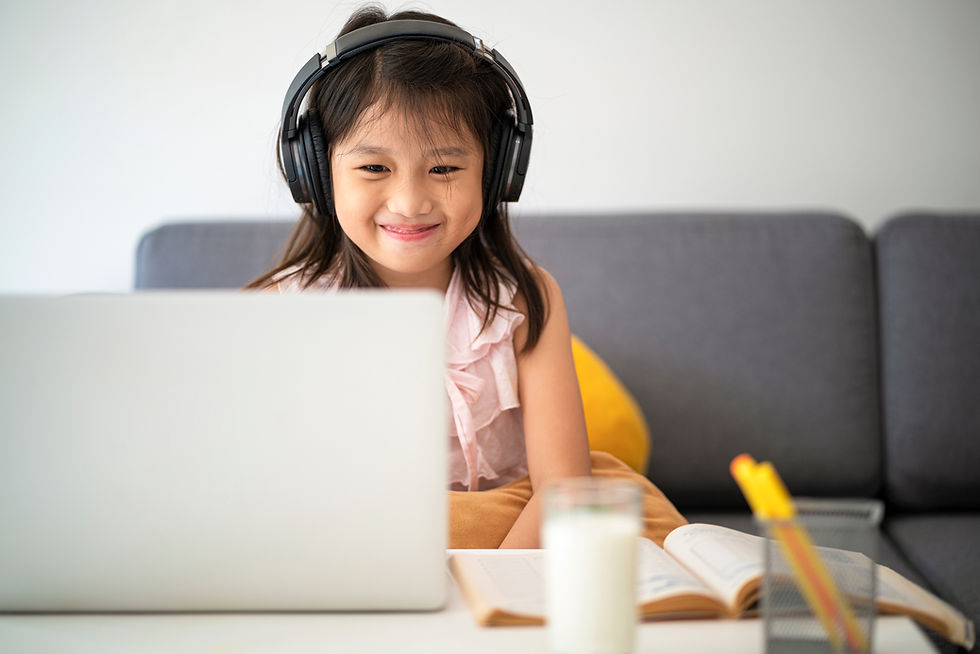
Venture funding for chip startups used to be rare. But in the last five years it’s more than doubled, thanks to AI. Gordon Moore famously predicted that every two years central processors units (CPUs) used inside laptops, desktops and servers would merely double their performance. But the raw computational power necessary to use Artificial Intelligence (AI) or Machine Learning (ML) has dwarfed everything else we use computer chips to accomplish in our day to day lives by an order of magnitude. This new pressing demand on specific type of computing chips has created a booming market for semiconductor startups for the first time in years. Nvidia has estimated that most machine learning or AI tasks have spurred a 25x increase in the need for processing power every two years, while one of the most advanced Natural Language Processing (NLP) models needs 275x the compute power every two years to work.
The jump in demand for computing horsepower helped make Nvidia the most valuable chip company in the U.S., as its graphics processing units (GPUs) and software stack can be harnessed for machine learning. But the boom has also created opportunities for a new breed of chip startups that are focused on making specialized chips for AI computation. Over the years, as AI workloads started to grow and expand, it gave the startups an opportunity to come in with purpose-built semiconductor chips that can meet the needs better than the general-purpose chips. Global sales of AI specific chips soared to $36B compared to 2020, with roughly half coming from specialized AI chips in mobile phones. Allied Market Research forecasts that sales number could rise to $195B by 2030.
VC Investment Interests
Semiconductor chips are expensive to make. The costs associated with new chip fabrication factories are measured by the tens of billions of dollars, and even companies that outsource fabrication to the likes of TSMC, UMC bear chip development costs that start at $30M-$40M and top out above $500M for the most-advanced processors. And chip design talent is scarce and costly. Today some of that has changed. Chips are still expensive to develop and require more capital from venture investors to get off the ground. But this no longer dissuades risk taking VC investors. With the advent of artificial intelligence use there has been a resurgence in semiconductor investing in startups. Many semiconductor startups that were founded 3-5 yrs. back, began to trial commercially viable AI chips in 2021 after years of research and development and several startups in the space have shipped trial chips to customers. As with just about any venture capital investment, the VCs writing the checks are looking for exponential returns and technology that can deliver exponential differences in performance. It’s not enough to be 30%-50% better, it needs to be 5x-10x better.
Success of AI is Tied to Infrastructure Layer
Artificial intelligence (AI) is bringing many changes to the enterprise, none of which is more vital to its success than infrastructure. For AI purpose, achieving far superior performance at the infrastructure level than the existing solution in the market typically means investing in startups developing chips and software around parallel processing, which takes on simpler tasks at far greater volume compared to a traditional processor that completes a single, usually more complex, calculation at a time. Changing nature of workloads – not just how they are generated and processed but how they apply to the operational goals – requires changes in the way raw data is handled, and this extends right down to the physical layer of the data stack. It doesn’t mean using the most-advanced chip manufacturing techniques, but rather rethinking how chips communicate with one another and how the data flows between the storage elements and compute elements within the chip itself. Large chip giants themselves already dump billions into annual research and development spending, including on parallel processing technology found in graphics chips made by AMD, Intel and Nvidia. VCs are funding companies that take a different approach, an idea that can’t be easily replicated by an incumbent semiconductor player. As example, some startups perform AI processing at a fraction of the power required to run the type of typical graphics chip that’s used in data centers. The way startups accomplish that wasn’t just via optimizing or tweaking circuits, it is by taking a whole new approach to computation.
Technologies and Architecture
AI is already changing the way infrastructure is being designed in the cloud and all the way out to the edge. But all hardware is interrelated in the enterprise, whether it sits in the data center, the cloud, or the edge, and this makes it difficult to simply deploy new platforms and put them to work. On a more fundamental level, basic hardware is becoming optimized to support AI workloads, and not just on the processor level. But it will take a coordinated effort to configure mixture of hardware to handle AI properly – and there isn’t likely to be one right way of doing it anyway. AI needs to reside on mix of purpose-built and general-purpose infrastructure if it is to bring real value to the business model. In a recent survey, lack of proper mix of infrastructure was cited as one of the primary drivers for failed AI projects, which continues to stymie development in more than two-thirds of organizations.
Much of the heavy lifting in AI model development and utilization is typically performed by front-end data conditioning tools. Hence choices over processor cores, acceleration technologies and memory cache may prove more consequential than the type of processor. Cutting-edge GPUs, for example, may be the solution-of-choice for advanced deep learning and natural language processing models, but several AI applications, some of them quite advanced, such as large-scale reinforcement learning are better-suited to the CPU. Memory architectures may also play a critical role in future AI platforms, because even the fastest chip in the world is of little use if it can’t access data, and for that you need high capacity and high bandwidth in the memory module. Research is turning toward AI-optimized memory solutions that could be used in tandem by CPUs, GPUs, and even custom ASICs, along with their own on-chip memory cores. A key aspect of this development revolves around the open Compute Express Link (CXL) that enables coherency between multiple memory cores.
Long Journey Towards Optimization
Infrastructure will not just be optimized around AI, but around all the different flavors of AI. Natural language processing (NLP) requires massive compute power to handle vast amounts of data. So, the network fabrics and the advanced software used to manage them will prove vital. The idea is not just to throw more raw compute power at AI but to streamline data flows, workflows and coordinate the activities of large and highly distributed data sets that AI utilizes. None of this will happen overnight. It took decades to bring data infrastructure to the state it’s in today, and it will take more years to tailor it to the needs of AI applications. But the incentive to achieve this is strong, and now that most enterprise infrastructure is being positioned by cloud providers as a core, revenue-generating asset, the need to be at the forefront of this infrastructure transition will likely drive all new IT deployments going forward. Despite all the changes that AI is poised to bring to the enterprise, one thing is clear: AI is all about vast amounts of data, and data lives in storage infrastructure. The only way to ensure that AI’s promises can be turned into reality is to create the right physical underpinnings to allow the intelligence to work its magic at the top layer.
/Service Ventures Team
Commentaires